Rethinking the energy supply chain with data and AI
The UK is pushing for 50 gigawatts of wind and 70 gigawatts of solar by 2030, and Germany is targeting 65% renewable electricity in the same timeframe. In the Nordics, Finland is aiming for 62% renewable energy by 2030, and Sweden 100% by 2040. Behind each of these targets lies a maze of interconnected decisions and dependencies that traditional approaches simply can’t manage.
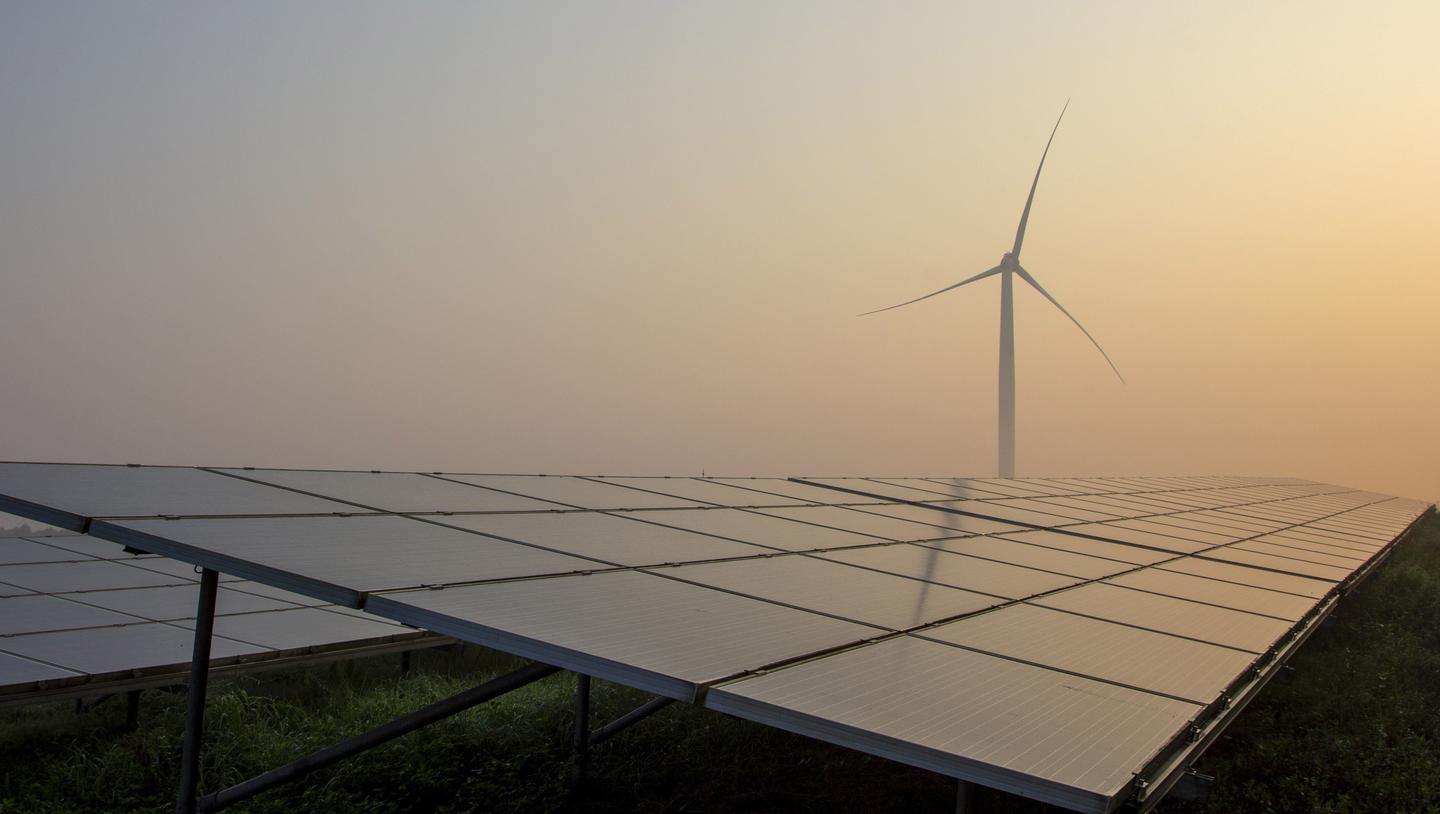
When I look at the challenges facing the energy sector today, what strikes me isn’t just the scale of the energy transition targets—it’s the sheer complexity of achieving them.
Last month, I spoke with several of our energy and AI experts to explore what’s possible with today’s technology. We looked beyond the usual PowerPoint-style view of digital transformation to see how data and AI can address real infrastructure challenges in the energy supply chain. Our discussion revealed some of the ways AI could transform approaches that haven’t fundamentally changed in decades.
Moving beyond traditional site selection
The parallels between energy site selection and challenges in other industries are clear. In forestry, for example, it’s all about optimizing land use based on multiple competing factors. Our experience with analyzing satellite imagery and environmental data in that sector has led to new possibilities for energy.
The traditional approach to developing sites for power generation follows a linear path—first, assess resource availability, then look at connection options, and finally examine planning constraints. But in today’s environment, with optimal sites increasingly scarce, this sequential approach no longer works. All factors must be considered simultaneously—and that’s where AI can transform the process.
The real opportunity lies in moving from static, point-in-time analysis to continuous optimization. Imagine a system that continuously processes new satellite imagery, updates grid capacity data, and integrates changes in planning frameworks. The technology exists; the challenge is developing models that reflect the way experienced developers evaluate sites.
A new approach to supply chain intelligence for energy infrastructure
The battery cost spike of 2021 was revealing—not just in its impact but in the energy industry’s blind spots. Many companies, even well-prepared ones, were caught off guard by focusing on the wrong indicators.
This mirrors what we’ve seen in other industries, where leading indicators of supply chain issues often come from unexpected sources—subtle changes in supplier operations visible in satellite imagery, for example, or regulatory shifts that cascade through supply chains. The next generation of supply chain intelligence will look radically different, moving from tracking past events to identifying patterns that predict future challenges.
Addressing the speed problem in energy infrastructure projects
Some of the most insightful conversations recently have been about project delivery timelines. While everyone recognizes the need to build energy infrastructure faster, adding more personnel often increases coordination challenges.
We’re developing what we call “high-speed project management” systems, a term inspired by one of our engineers who likened the task to “running an industrial process at software development speed.” This approach focuses on accelerating decision-making in complex projects with thousands of interdependent tasks and dozens of stakeholders. Here, the true bottleneck is rarely the physical work itself—it’s the pace of decision-making when things don’t go as planned.
Rethinking predictive maintenance with acoustic analysis
Most of us in the industry have seen predictive maintenance presentations over the years, but acoustic analysis presents another angle worth exploring. Although not completely new, AI-powered sound analysis in industrial settings could detect equipment issues weeks before they surface in other metrics. While still in the exploratory phase, the potential for acoustic analysis goes beyond technology itself. By identifying issues before they become significant, maintenance teams can focus on the areas that need attention most urgently.
This is where AI could be truly transformative—not by replacing human expertise but by focusing maintenance teams’ efforts where it matters most. Effective predictive maintenance systems do more than issue alerts; they help teams understand why predictions are made and provide actionable insights for addressing potential failures.
Looking forward: Applying AI to high-impact energy challenges
The path forward isn’t about technology for its own sake but about fundamentally new ways of managing energy infrastructure. Our work in other sectors shows that the biggest impact comes from solving specific, high-value challenges rather than broad digital transformation. The teams that succeed in this transition will not necessarily be those with the largest technology budgets or the most advanced AI. Instead, they will be those that thoughtfully apply these tools to their most pressing challenges, learning from the results and expanding gradually from there.
The energy transition demands unprecedented speed and scale. By learning from other industries and applying new technologies strategically, we can help make this transition possible.
Join our upcoming webinar: Rethinking the Energy Supply Chain with Data & AI To dive deeper into these topics, join us for our upcoming webinar, Rethinking the Energy Supply Chain with Data & AI. We’ll explore the current state of the energy supply chain, discuss the biggest pain points, and reveal how data and AI can unlock transformative solutions previously out of reach. Whether you’re navigating these challenges or looking to innovate, this webinar will provide actionable insights and tools for making a sustainable impact.
- David MitchellChief Growth Officer